使用TensorFlow进行图像分类
💓 博客主页:瑕疵的CSDN主页
📝 Gitee主页:瑕疵的gitee主页
⏩ 文章专栏:《热点资讯》
使用TensorFlow进行图像分类
- TensorFlow简介
- 环境搭建
- 安装TensorFlow
- 数据准备
- 下载数据集
- 模型构建
- 编译模型
- 训练模型
- 评估模型
- 预测新图像
- 模型优化
- 数据增强
- 使用预训练模型
- 总结
TensorFlow是一个由Google开发的开源机器学习框架,广泛应用于各种深度学习任务。本文将详细介绍如何使用TensorFlow进行图像分类,包括环境搭建、数据准备、模型构建、训练过程、评估模型、预测新图像以及模型优化等内容。
TensorFlow是一个端到端的开源机器学习平台,支持多种编程语言,包括Python、C++和Java。它提供了强大的工具和库,使得构建和训练复杂的机器学习模型变得简单。 在开始之前,确保你的环境中已安装Python和pip。pip install tensorflow
为了进行图像分类,我们需要准备训练数据集。常用的数据集包括MNIST、CIFAR-10和ImageNet等。
import tensorflow as tf
from tensorflow.keras.datasets import cifar10(train_images, train_labels), (test_images, test_labels) = cifar10.load_data()# 归一化图像数据
train_images, test_images = train_images / 255.0, test_images / 255.0
使用Keras API构建一个卷积神经网络(CNN)模型。
from tensorflow.keras import layers, modelsmodel = models.Sequential([layers.Conv2D(32, (3, 3), activation='relu', input_shape=(32, 32, 3)),layers.MaxPooling2D((2, 2)),layers.Conv2D(64, (3, 3), activation='relu'),layers.MaxPooling2D((2, 2)),layers.Conv2D(64, (3, 3), activation='relu'),layers.Flatten(),layers.Dense(64, activation='relu'),layers.Dense(10)
])model.summary()
在训练模型之前,需要编译模型,指定损失函数、优化器和评估指标。
model.compile(optimizer='adam',loss=tf.keras.losses.SparseCategoricalCrossentropy(from_logits=True),metrics=['accuracy'])
使用训练数据集训练模型。
history = model.fit(train_images, train_labels, epochs=10, validation_data=(test_images, test_labels))
评估模型在测试数据集上的性能。
test_loss, test_acc = model.evaluate(test_images, test_labels, verbose=2)
print(f'\nTest accuracy: {test_acc}')
使用训练好的模型对新图像进行分类。
import numpy as np# 假设有一个新图像 new_image
new_image = test_images[0]
new_image = np.expand_dims(new_image, axis=0)predictions = model.predict(new_image)
predicted_class = np.argmax(predictions[0])
print(f'Predicted class: {predicted_class}')
可以通过调整超参数、增加数据增强和使用预训练模型等方式来优化模型。
from tensorflow.keras.preprocessing.image import ImageDataGeneratordata_augmentation = ImageDataGenerator(rotation_range=15,width_shift_range=0.1,height_shift_range=0.1,horizontal_flip=True,vertical_flip=False
)train_generator = data_augmentation.flow(train_images, train_labels, batch_size=32)history = model.fit(train_generator, epochs=10, validation_data=(test_images, test_labels))
from tensorflow.keras.applications import VGG16base_model = VGG16(weights='imagenet', include_top=False, input_shape=(32, 32, 3))model = models.Sequential([base_model,layers.Flatten(),layers.Dense(64, activation='relu'),layers.Dense(10)
])model.compile(optimizer='adam',loss=tf.keras.losses.SparseCategoricalCrossentropy(from_logits=True),metrics=['accuracy'])history = model.fit(train_images, train_labels, epochs=10, validation_data=(test_images, test_labels))
通过本文,你已经学习了如何使用TensorFlow进行图像分类。我们介绍了TensorFlow的基本概念、环境搭建、数据准备、模型构建、训练过程、评估模型、预测新图像以及模型优化等内容。掌握了这些知识,将有助于你在实际工作中更好地利用TensorFlow来构建高效、准确的图像分类模型。
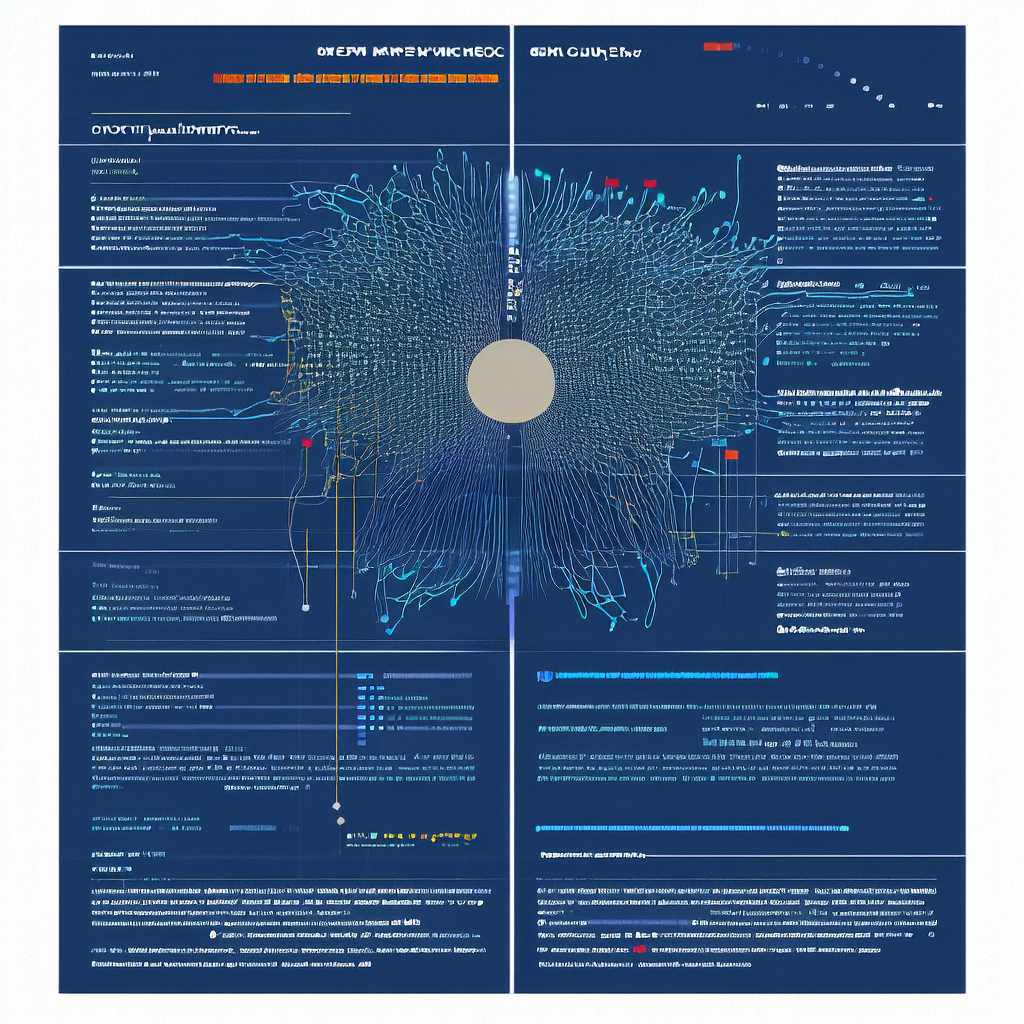
使用TensorFlow进行图像分类可以显著提高模型的准确性和泛化能力。