旷视科技ShuffleNetV1代码分析[pytorch版]
一、前述
旷视科技针对于ShuffleNet系列网络在GitHub网站上已开源,其链接:https://github.com/megvii-model/ShuffleNet-Series
在这个系列中,包括了ShuffleNetV1/V2网络,如下图所示。
我们点开ShuffleNetV1文件夹,如下图所示。
- ShuffleNetV1文件夹中有五个文件,分别为:README.md、blocks.py、network.py、train.py、utils.py文件。
- 其中,blocks.py中的代码是ShuffleNetV1的基本模块;
- network.py 中的代码是 blocks.py 中基本模块堆叠出来的 ShuffleNetV1 网络;
- train.py 中是训练 ImageNet 数据集图像分类的训练代码;
- utils.py 是一些常用的函数。
旷视科技GitHub网站给出的ShufflNetV1网络的结果,如下表所示:
二、代码分析
2.1 blocks.py(ShuffleNetV1 Unit)
我们先来回顾以下ShuffleNetV1 Unit,如下图(b)、图(c)所示。
图(b)表示的是stride=1的ShuffleNetV1 Unit,在该基本单元中,右侧被称为主分支,在该主分支中:
①先1×1GConv(group pointwise convolution)降维,第一个红色模块;
②然后channel shuffle,蓝色模块;
③再然后3×3DWConv(depthwise convolution),绿色模块;
④然后再1×1GConv升维,第二个红色模块。
图(c)表示的是stride=2 的ShuffleNetV1 Unit,在该基本单元中,右侧被称为主分支,在该主分支中:
①先1×1GConv(group pointwise convolution)降维,第一个红色模块;
②然后channel shuffle,蓝色模块;
③再然后3×3DWConv(depthwise convolution),绿色模块;
④然后再1×1GConv升维,第二个红色模块。
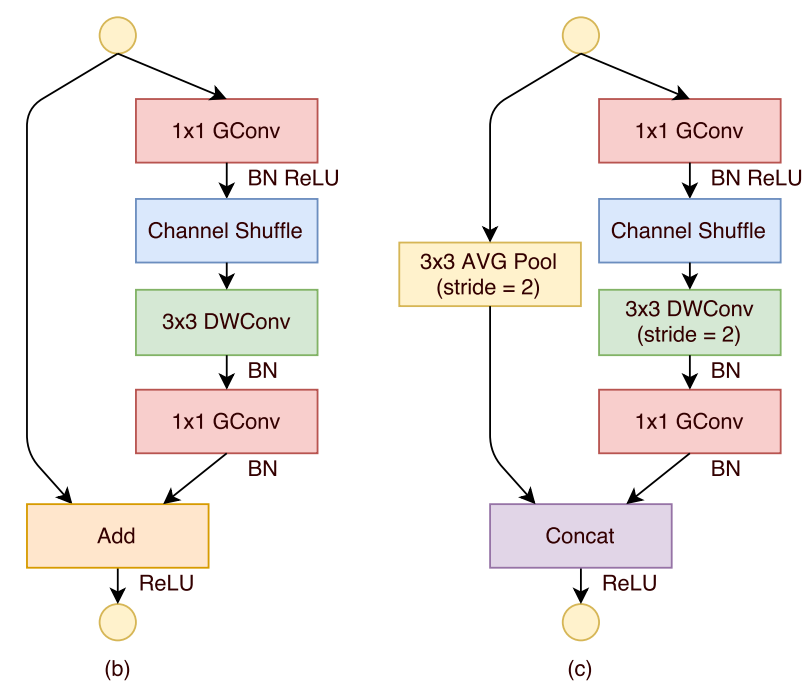
(b)stride = 1; (c)stride = 2
ShuffleNetV1网络基本模块的总体代码如下所示,该代码包括了:stride=1的基本单元构建、stride=2的基本单元构建、channel shuffle(通道重排)操作。
# blocks.py
import torch
import torch.nn as nn
import torch.nn.functional as Fclass ShuffleV1Block(nn.Module):def __init__(self, inp, oup, *, group, first_group, mid_channels, ksize, stride):super(ShuffleV1Block, self).__init__()self.stride = strideassert stride in [1, 2]self.mid_channels = mid_channelsself.ksize = ksizepad = ksize // 2self.pad = padself.inp = inpself.group = groupif stride == 2:outputs = oup - inpelse:outputs = oupbranch_main_1 = [# pwnn.Conv2d(inp, mid_channels, 1, 1, 0, groups=1 if first_group else group, bias=False),nn.BatchNorm2d(mid_channels),nn.ReLU(inplace=True),# dwnn.Conv2d(mid_channels, mid_channels, ksize, stride, pad, groups=mid_channels, bias=False),nn.BatchNorm2d(mid_channels),]branch_main_2 = [# pw-linearnn.Conv2d(mid_channels, outputs, 1, 1, 0, groups=group, bias=False),nn.BatchNorm2d(outputs),]self.branch_main_1 = nn.Sequential(*branch_main_1)self.branch_main_2 = nn.Sequential(*branch_main_2)if stride == 2:self.branch_proj = nn.AvgPool2d(kernel_size=3, stride=2, padding=1)def forward(self, old_x):x = old_xx_proj = old_xx = self.branch_main_1(x)if self.group > 1:x = self.channel_shuffle(x)x = self.branch_main_2(x)if self.stride == 1:return F.relu(x + x_proj)elif self.stride == 2:return torch.cat((self.branch_proj(x_proj), F.relu(x)), 1)def channel_shuffle(self, x):batchsize, num_channels, height, width = x.data.size()assert num_channels % self.group == 0group_channels = num_channels // self.groupx = x.reshape(batchsize, group_channels, self.group, height, width)x = x.permute(0, 2, 1, 3, 4)x = x.reshape(batchsize, num_channels, height, width)return x
我们一步一步做好乐高积木然后将这些乐高积木拼装起来,如下:
图(b)主分支代码如下:
图(c)主分支代码如下:
图(c)侧分支代码如下:
channel shuffle代码:
做好乐高积木之后,我们在forward函数中开始搭建这些乐高积木,如下所示:
2.2 networks.py (ShuffleNetV1网络架构)
ShuffleNetV1网络架构:
ShuffleNetV1 网络架构代码:
import torch
import torch.nn as nn
from blocks import ShuffleV1Blockclass ShuffleNetV1(nn.Module):def __init__(self, input_size=224, n_class=1000, model_size='2.0x', group=None):super(ShuffleNetV1, self).__init__()print('model size is ', model_size)assert group is not Noneself.stage_repeats = [4, 8, 4]self.model_size = model_sizeif group == 3:if model_size == '0.5x':self.stage_out_channels = [-1, 12, 120, 240, 480]elif model_size == '1.0x':self.stage_out_channels = [-1, 24, 240, 480, 960]elif model_size == '1.5x':self.stage_out_channels = [-1, 24, 360, 720, 1440]elif model_size == '2.0x':self.stage_out_channels = [-1, 48, 480, 960, 1920]else:raise NotImplementedErrorelif group == 8:if model_size == '0.5x':self.stage_out_channels = [-1, 16, 192, 384, 768]elif model_size == '1.0x':self.stage_out_channels = [-1, 24, 384, 768, 1536]elif model_size == '1.5x':self.stage_out_channels = [-1, 24, 576, 1152, 2304]elif model_size == '2.0x':self.stage_out_channels = [-1, 48, 768, 1536, 3072]else:raise NotImplementedError# building first layerinput_channel = self.stage_out_channels[1]self.first_conv = nn.Sequential(nn.Conv2d(3, input_channel, 3, 2, 1, bias=False),nn.BatchNorm2d(input_channel),nn.ReLU(inplace=True),)self.maxpool = nn.MaxPool2d(kernel_size=3, stride=2, padding=1)self.features = []for idxstage in range(len(self.stage_repeats)):numrepeat = self.stage_repeats[idxstage]output_channel = self.stage_out_channels[idxstage+2]for i in range(numrepeat):stride = 2 if i == 0 else 1first_group = idxstage == 0 and i == 0self.features.append(ShuffleV1Block(input_channel, output_channel,group=group, first_group=first_group,mid_channels=output_channel // 4, ksize=3, stride=stride))input_channel = output_channelself.features = nn.Sequential(*self.features)self.globalpool = nn.AvgPool2d(7)self.classifier = nn.Sequential(nn.Linear(self.stage_out_channels[-1], n_class, bias=False))self._initialize_weights()def forward(self, x):x = self.first_conv(x)x = self.maxpool(x)x = self.features(x)x = self.globalpool(x)x = x.contiguous().view(-1, self.stage_out_channels[-1])x = self.classifier(x)return xdef _initialize_weights(self):for name, m in self.named_modules():if isinstance(m, nn.Conv2d):if 'first' in name:nn.init.normal_(m.weight, 0, 0.01)else:nn.init.normal_(m.weight, 0, 1.0 / m.weight.shape[1])if m.bias is not None:nn.init.constant_(m.bias, 0)elif isinstance(m, nn.BatchNorm2d):nn.init.constant_(m.weight, 1)if m.bias is not None:nn.init.constant_(m.bias, 0.0001)nn.init.constant_(m.running_mean, 0)elif isinstance(m, nn.BatchNorm1d):nn.init.constant_(m.weight, 1)if m.bias is not None:nn.init.constant_(m.bias, 0.0001)nn.init.constant_(m.running_mean, 0)elif isinstance(m, nn.Linear):nn.init.normal_(m.weight, 0, 0.01)if m.bias is not None:nn.init.constant_(m.bias, 0)if __name__ == "__main__":model = ShuffleNetV1(group=3)# print(model)test_data = torch.rand(5, 3, 224, 224)test_outputs = model(test_data)print(test_outputs.size())
分析:
asset函数:
张量的连续性:https://blog.csdn.net/m0_48241022/article/details/132804698
如何理解张量、张量索引等:https://blog.csdn.net/m0_48241022/article/details/132729561
torch.nn.Conv2d函数:
torch.nn.BatchNorm2d函数:
torch.nn.ReLU函数:
torch.nn.AvgPool2d函数:
torch.nn.Linear函数:
torch.nn.Sequential函数:
torch.cat函数:
permute函数: